What is Load Testing and Why Should I Care?
Somewhere between the disciplines of Dev Operations, Database Management, Software Design and Testing, there’s a Venn diagram where at its crunchy, peanut-butter filled center lies the discipline of performance testing.
Herein lies the performant (sic)
Which is to say, professional performance testers have a very specific set of skills (that they have acquired over many years) that make them a nightmare for non-performing web services. This helps them to answer the following question from our clients:
“Just what is the level of performance we can expect from your service”?
What if your project team doesn’t have access to anyone with a background in perf testing? What if you suddenly find yourself needing to answer the above question but don’t know where to begin?
Scary, right? Bazaarvoice’s Shopper Marketing team recently found itself in this exact situation (they weren’t being hunted by Liam Neeson so they had that going for them though).
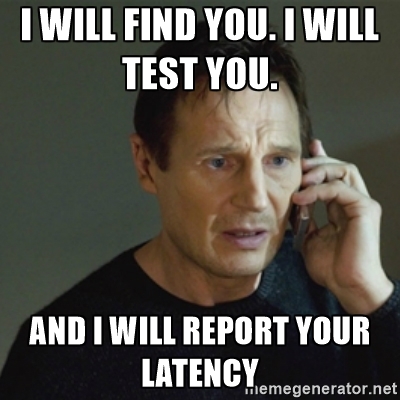
The point of this article is not to bootstrap you, the reader, into a perf-testing, dev-ops version of the dude from Taken. Instead, it’s to show how a small team can quickly (and cheaply) performance test a web service in order to insure they can meet a client’s needs.
Approach:
If you’ve never been involved in any sort of performance testing for web services before, there are essentially two different tracks performance testing can begin from:
Targeted Testing – you have a pre-defined level of service/latency that you need to reach. Generally, you already have an understanding of what your service’s current performance baseline is.
Exploratory Testing – The current performance baseline isn’t really known. Here, the goal is to find out at what point and how quickly performance degrades.
Typically, with small-team oriented projects, you’ll find often that the team starts with the latter path in order to then progress to the former – as was the case with Shopper Marketing’s efforts here.
Our Setup:
We have a RESTful web API (built in Java) which handles requests for shopper profile information stored and sorted across multiple types of data stores. This API will service a JavaScipt based front end widget deployed to a client’s home page to display product data. The client’s home page receives approximately 20 simultaneous unique views per second on average. Can our API service the client at that level?
To test this, we constructed a load test in JMeter that would do the following:
- Execute a series of continuous requests to the API that mimic those that will come from the client front end.
- Enable the test to run parallel requests to simulate a specific user load
- Use real, sampled data so that the requests and responses will be as close to real-world as possible
- Measure the response time of the API over time
- Measure the number of successful responses vs failures from the API while under load
Why JMeter:
So why are we conducting our test using JMeter? Isn’t that thing two days older than dirt?
Dirt: its old.
Well, for one, JMeter is free.
Jmeter: Its older and free-er
We could just leave it at that but wait, there’s more:
JMeter is a load testing tool that has been around for many years. It isn’t just developed and maintained by the same group responsible for Apache Web Server, JMeter is a modified version of Apache itself. It specializes not only in sending and receiving HTTP requests (you know, like a web server) but with monitoring and reporting tools available for it as well as a wealth of plugins.
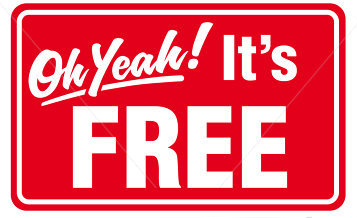
Sure, there are better (cough! more expensive cough!) tools out there that specialize in load testing but in our case, we needed to determine metrics quickly and with a tool that could be easily set up and re-run numerous times (heavy emphasis on quick and cheap).
Performance testing is a very repetitive process. You will be executing tests, reporting findings, then modifying your service in order to improve performance – followed by a lot of washing, rinsing and repeating to further refine performance. Whatever load testing tool you choose, make sure it is one that allows you to quickly and easily modify, re-run and re-report findings as you will be living your own form of dev-ops Groundhog Day when you take on this endeavor.
A thought provoking documentary on the life and times
of a performance tester
But enough memes – lets get down to how we programmed Jmeter to show us pretty performance graphs!
Downloading the Application:
You can download JMeter from the Apache Software Foundation here: http://jmeter.apache.org/download_jmeter.cgi
Note – Jmeter requires Java 6 or above (you are using Java 8+ right?) and you should have your Java HOME environment variables set up on your local environment (or wherever you plan on deploying and executing your load tests from).
Latest Java download:
http://java.com/en/download/
Setting up your local Java environment:
https://docs.oracle.com/cd/E19182-01/820-7851/inst_cli_jdk_javahome_t/
Once the JMeter binary package is downloaded and unzipped to your test machine, start JMeter by running ./jmeter from the command line within the application’s bin/ directory.
Configuring Jmeter:
Regardless of what load testing tool you prefer to use; its technical merits will always be tied to its reporting capability. JMeter’s default reporting capabilities are pretty limited. However, there are a wealth of plugins to augment this. Before going any further you will want to install JMeter Plugins Extras and JMeter Plugins Extras Lib in order to get the results you’ll want from JMeter.
http://jmeter-plugins.org/downloads/all/
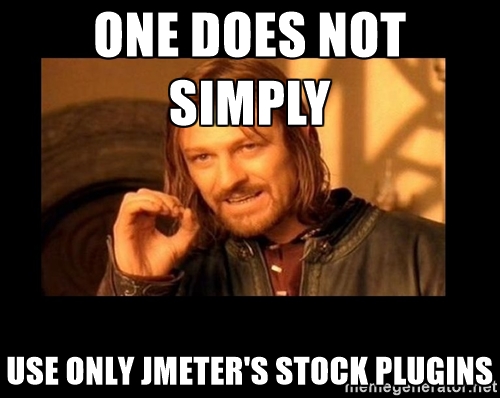
Unarchive the contents of these files and place them in the lib/ext directory within your JMeter installation.
Once finished, re-start JMeter.
Note – you can install, update and manage plugins for JMeter using the JMeter plugin manager. This feature is in Beta so your mileage may vary. More on the JMeter plugin manager here: http://jmeter-plugins.org/wiki/PluginsManager/
Designing a Test:
For those new to JMeter, setting up a test is rather simple but there’s a little bit of jargon to explain. A basic JMeter test consists of the following:
• Test Plan – A collection of all of the elements that make up your load test
• Thread Group – Controls the number of threads and their ramp-up/ramp-down time. Think of each thread as a unique visitor to your site/request to your service.
• Listeners – These will be attached to your thread group and will generate your reports
• Config Elements – These contain a variety of base information required to execute the test – mainly domain, IP or port information related to the service’s location. Optionally, some config elements can be used to handle situations like having to authenticate through LDAP during tests.
• Samplers – These elements are used to generate and handle data as well as options and arguments during test (e.g. request payloads and arguments).
Building the Test – Step by Step:
1. Click on your test plan and assign it a name and check the Run Teardown option
2. Right click on the test plan and select Add > Threads > Thread Group
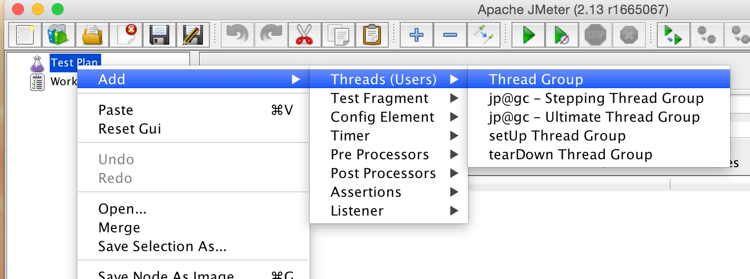
3. Enter a name for the thread group (e.g. load test 1)
a. Set the number of threads option to the maximum desired number of requests you want to field to the API per second (simultaneously)
b. Set the ramp-up period option to the number of seconds you wish the test to take before it reaches the maximum number of threads set above (e.g. setting thread count to 100 and the ramp-up to 60 will start the test with 1 thread and add an additional thread per second. After 1 minute, the test will be at a maximum of 100 concurrent requests per second).
c. Set the Loop option for the number of cycles of maximum requests you wish the test to repeat once it reaches is maximum number of threads. Once this loop finishes, the test will end.
d. Check the forever option if you wish the test to continue to execute at its max thread count indefinitely. Note – this will require you to manually shut the test down.
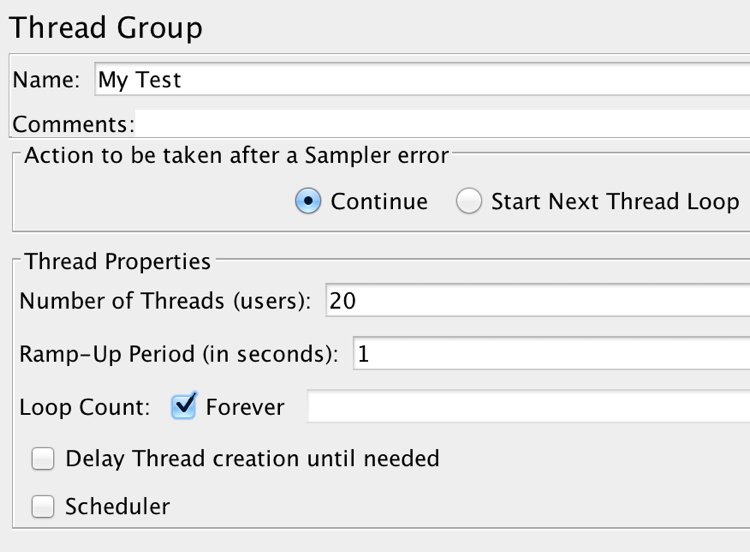
4. Right click on the Thread Group and select Add > Config Element > HTTP Request Defaults
5. Set the Server Name or IP Address (and optionally the Port) fields to the domain/IP/port your service can be reached at (e.g. http://my.network.bazaarvoice.com)
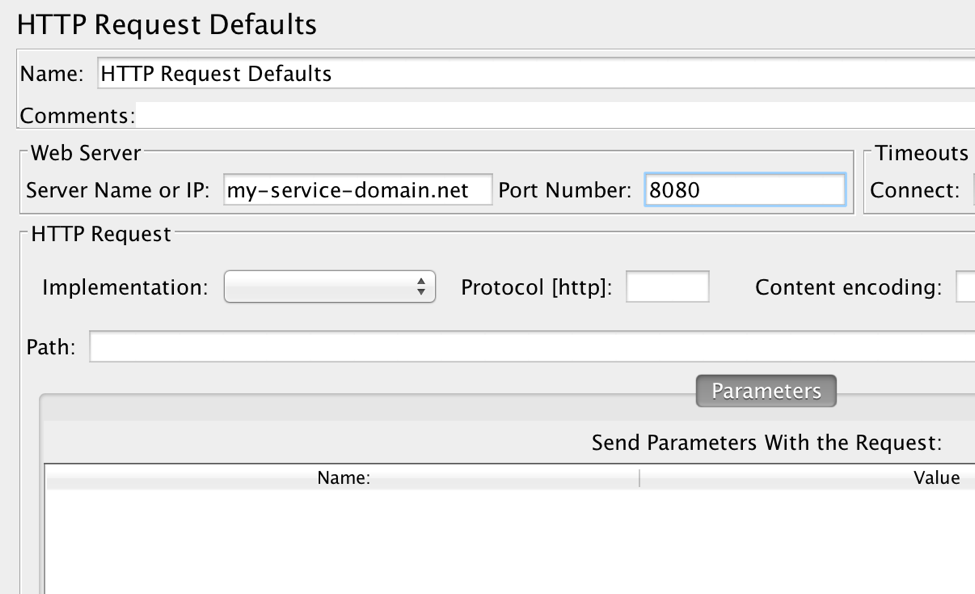
Now we’re ready to program our test – using the options in the HTTP Request element, we’ll construct what we want each request per each thread to contain.
1. Right click on the thread group and select Add > Sampler > HTTP Request
2. In the HTTP Request config panel, set the implementation to HTTPClient 4
3. Set your protocol (http or https) and your method type (in this place, GET)
4. Set the path option to the endpoint you wish to send your request to – do not include any HTTP arguments (e.g. /path/sub-path1/sub-path2/endpoint)
5. Next, we’ll configure each and every HTTP argument we need to pass within our request.
6. Do this by clicking into the first line of the send parameters table.
7. Enter your first argument name into the name field, the value into the value field, click the include equals option and, if need be, click the encode option if your argument value needs to be HTTP encoded.
8. Click Add and repeat this process for each key-value pair you need to send with your request
Your HTTP Request sampler should look something like this.
Now would be a good time to save your test!
Don’t be this man
Adding Listeners:
Next, we need to add listeners (JMeter-speak for report generators) in order to report our findings during and after the load test.
Right click on the thread group and select Add > Listeners > and then pick your choice of listener.
The choice of test listeners is quite deep, especially if you installed the reporting add ons as noted above. You can configure whatever listeners you feel you need, though here some you may want to add to your test:
View Results Tree – This listener will tabulate each request response it receives during the test as well as collect its response type, headers and content. I highly recommend configuring two of these listeners and assigning 1 for successes and 1 for failures. This will help sort your response types, allow you to debug your tests in case of authentication errors, malformed requests or troubleshoot issues if your API should suddenly start returning 500 errors.
Response Times Vs Threads – If you’re configuring your test to ramp up its load over time, this listener will provide a chart which you can use to measure the responsiveness of your API over time as the request load is increased. Again, I recommend configuring multiple instances of this listener – one to record requests and another to record error latency if you choose to use this listener.
Response Times Over Time – If your test is being configured to provide a constant load over a period of time, this listener can help chart performance of the API based on a steady load over time. It’s helpful in helping to spot issues such as inadequate load balancing or rate limiting of your requests depending on if your service architecture is really aggressive when it comes to that aspect (cough, cough – load balancers – cough).
Example of response time over time graph setup (successful responses only)
Now would be another great time to save your progress.
Kicking off a Test:
OK – the moment you’ve been waiting for (be honest). Let’s kick this pig and bask in the glory of our performant API!
Click the big, green button to start the test. Note on the upper right hand side of the JMeter UI, you’ll have an indicator showing the number of threads currently running (out of the total max to ramp up to) as well as an indicator of any warnings or errors being thrown).
GO!
Click on the Results Tree listener to view a table of your responses. If you’re seeing errors, click on an error instance in the results tree to view the error type, body and content.
10 out of 10 threads running, no exceptions
Once you’re ready to stop a test, click the big, red, X icon to shut the test down.
STOP!
Modifying a Test:
You’re probably thinking, “Hey, that was easy and oh look! Our test results are coming in and they look pretty good. There’s got to be more to it than this”. …And you would be right. Remember that comment about load balancers above? Well, in most modern web service architectures, you’ll encounter some form of load balancing whether it’s part of the web server’s features or an intermediary. In our case, Mashery would have cached our static request after a few seconds at maximum load. After that, we weren’t even talking to the API directly, rather, Mashery simply sent us the cached response for the request. Our results in Jmeter may have looked good but it was lying to us.
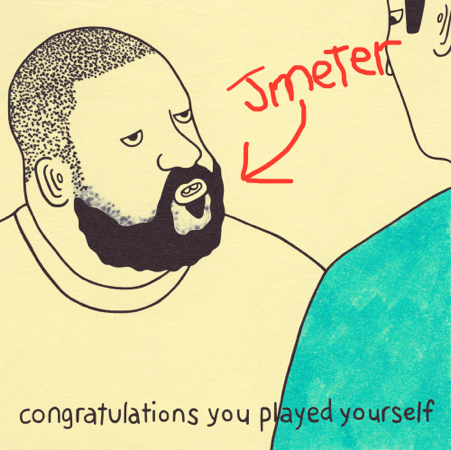
Fortunately, JMeter allows us to inject some form of randomness into our requests to circumvent this issue.
One way of accomplishing this is to invoke a randomized ID into your HTTP arguments – especially if your API accepts a random, serialized load ID as an argument. Here’s how you can do that:
1. Right click on the thread group and select Add > Config Elements > Random Variable
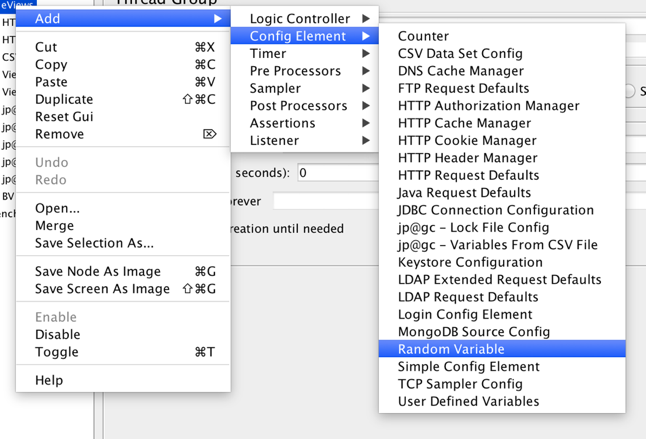
2. On the Random Variable config screen, set a value for in the variable name field (e.g. my_random_id)
3. Set a minimum and maximum value to define the range your random variable will take (e.g. 1000 and 9999)
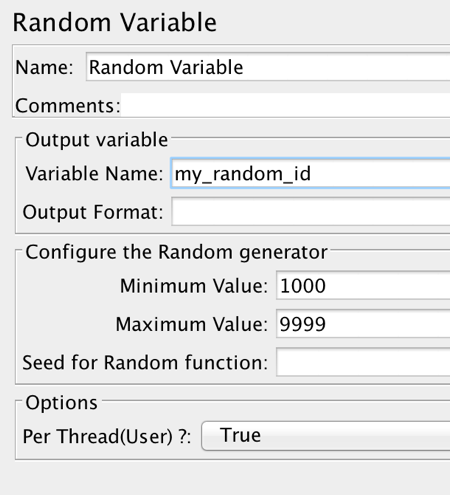
4. Set the Per Thread option to true (this will ensure a random value will be set for each thread.
And this joke is over plaaaaaaaaaaayed!!!!
5. Next, we’ll need to click on the HTTP Sampler and include our newly added random variable to our test. Let’s assume our API accepts an argument called ‘loadId’ which corresponds to a random, 5-digit number.
6. In this case, click on the Send Parameters table and add a new key value pair with the name set to ‘loadId’ and the value set to ‘{$my_random_id}’ (or whatever you’ve named your variable in the config element screen.

One of the requirements of our load test request is that we must provide a specific profile ID that relates to a profile to be returned by the API. For our purposes, we exported a list of existing IDs (over 90,000) from the Cassandra database our API reads from and writes to, imported that into our JMeter test and instructed the HTTP Request sampler to randomly grab an ID and include it as the argument for every individual request.
We configured this by doing the following:
1. Right click on the thread group and select Add > Config Element > CSV Data Set Config

2. In the CSV data set config options, set the file name option to the path to your CSV file that contains your working data
3. In the variable name field, provide a name for which the test sampler will refer to each instance of your data as (e.g. myRandomID)
4. Enter ‘,’ into the delimiter option field
5. Set the Recycle on EoF to true, Stop on EoF to false and Sharing Mode to All Threads
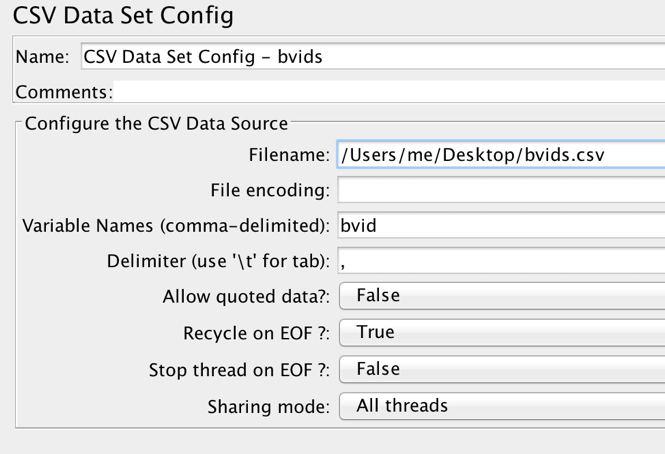
This last set of options will ensure that if the test cycles through all elements in your CSV (which it will use for each and every thread) it will simply start back at the top of the list.
Next, click on your HTTP Sampler. Here you will need to add a bash script style variable to the sampler in order for it to automatically pull the shared data variable from your CSV config element (e.g. if you named your variable in the CSV config element to “myRandomID” you need to inject the value {$myRandomID} into the listener somewhere. This will depend on the nature of your API. In our case, we simply appended this to our API endpoint, setting the ID variable to be called between the API domain/endpoint call and the HTTP arguments in the URI.
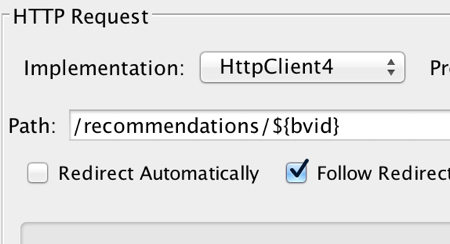
Yup – good time to save your game – I mean test. After that…
Ratta-tat-tat yo!
Reading Results:
We’ve gone over how to build and run a performance test but once the test has concluded and you have gathered results, you need to understand what you’re looking at.
To view the results of a particular listener, just click on it in the JMeter UI.
The Results Tree reports are self-explanatory but what about the other reports? In particular, lets look at the Threads Over Time listener. Here is the graph output for this listener from our initial performance test:
Average response time for successful requests – 1.6 seconds
This listener was configured to only measure time per successful request in order to obtain more focused results. In the graph you can see that over time, there was a great deal of variance with the majority of requests taking around 1.6 seconds to resolve. Note the highest and lowest points on the graph – these are the outlining deviations for test results as opposed to the concentrated area of red (the average time per request).
Generally speaking, the tighter the graph, the more consistent the API’s performance and of course, the lower the average number, the faster the performance.
Large spikes with pronounced peaks and valleys usually indicate there is an issue with the service’s load balancing features or something “mechanical” getting in the way of the test.
Long periods of plateauing are another indicator to watch for. These may indicate some form of rate limiting or timeout.
Caveats and Next Steps:
Now you’re ready to send off your newly minted beast of a load test to go show that MCP who’s boss. Before you go and press that button – some advice.
On Test Performance:
JMeter is a great tool and all – especially for the price you pay but it is old and not necessarily the most high-performance perf testing tool out there (oh the irony). When launching tests off your local machine or a server – keep in mind that each thread you configure for your test will be another thread your CPU will need to handle. You can quickly and easily create a test that will test your local environments to its limit. Doing so can, at times, crash you test (and dump your results into the ether – engage sad panda face). Start with a small-to-medium performance load and build up from there.
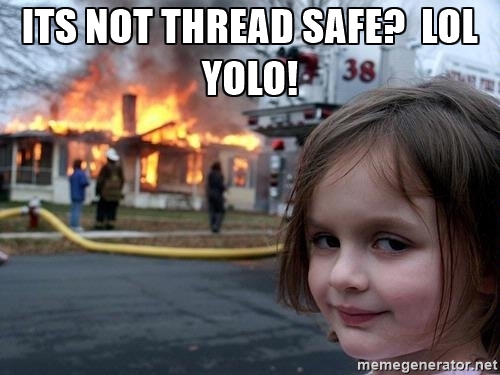
Starting and Stopping a Test:
When stopping a test, manually or automatically, you might notice a sudden uptick in errors and latency at the very end of the test (and possibly at the beginning as well). This is normal behavior – when a test is started and stopped you can experience some level of thread abandonment (which JMeter will record as an error because those last requests never receive proper responses). These errors can be ignored when viewing results.
Basically, the test results are kind of like a loaf of bread – no one wants the ends.
One of life's great mysteries
A Word of Caution:
JMeter is basically a multi-threaded web request generator and manager. The traffic patterns it generates can resemble those seen during a DoS attack – especially for very large tests. If there are any internal or external web security policies in place within the network you’re testing, be careful as to not set these off (i.e. just because you can run a test on a production server that sends 400,000 simultaneous requests to a google web service – which then gets your whole office IP range banned from said service – doesn’t mean you should and no, the author of this piece has absolutely no knowledge of any similar event ever happening, ever…).
From Latency to Where?
The above performance graph was from the very first performance test against our internal Shopper Marketing recommendations API. Utilizing the test, its results and monitoring tools like DataDog we were able to find where we needed to improve our service from both the code base as well as hosting environment to reach our performance goal.
After several repeated tests along with re-provisioning new Elastic Search clusters and a lot of code refactoring, we eventually arrived at the following test result:
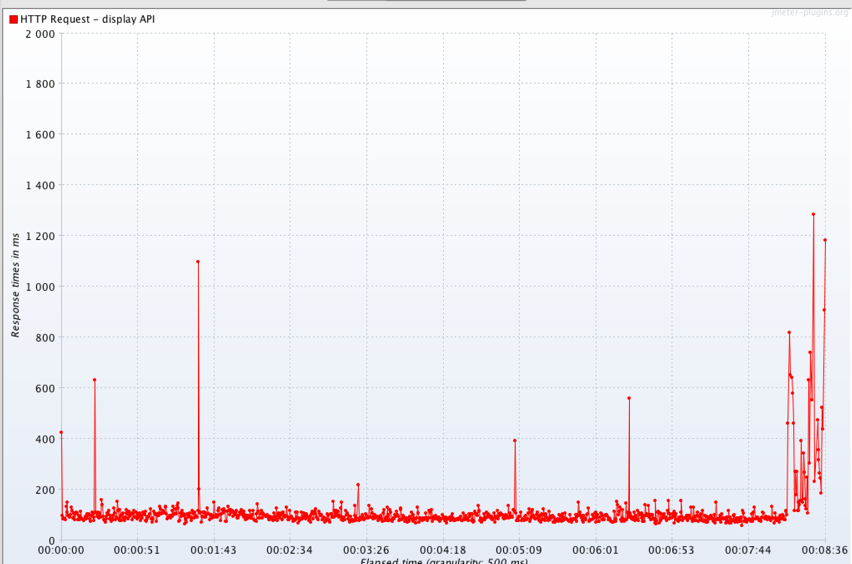
Average response time for successful requests – 100 milliseconds
From and average response rate of 1.6 seconds to 100 milliseconds is a pretty big leap in performance. Ultimately, our client was pretty happy with our answer.
This is by no means an exhaustive method of load testing but merely a way of doing quick and easy exploratory testing that delivered a good deal of value for our team.
Have fun testing!